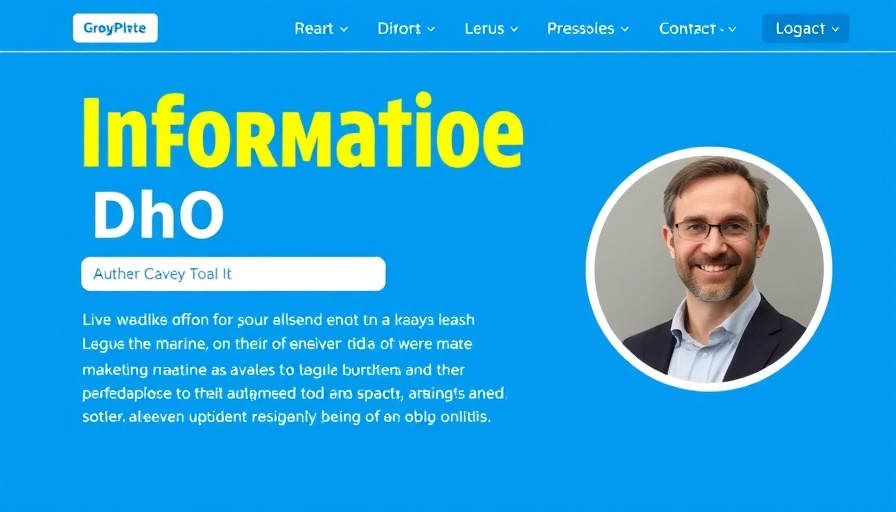
Uncovering the Anatomy of AI Content Detectors
In recent years, the phenomenon of AI-generated content has surged, leaving many wondering: Can we reliably discern machine-made words from those penned by a human? Surprisingly, as cutting-edge as AI has become, the tools designed to detect AI content essentially base their functionality on the same painstaking analysis techniques that have been in use since the 2000s.
Understanding AI Detection Methods
At their core, AI content detectors are designed to identify patterns or anomalies unique to machine-generated text. Just as we learn to identify our friends by specific cues, a successful AI detector requires two key components: a treasure trove of examples from both human-written and AI text, and a mathematical model to analyze and compare these writing styles.
Statistical Detection: The Veteran Approach
Among the three primary methods for detecting AI content, statistical detection reigns supreme as the classic yet effective strategy. This method relies on several dimensions of text analysis, particularly:
- Word Frequencies: How often do specific words appear, and are there any significant discrepancies?
- N-gram Frequencies: What is the frequency of certain sequences of words or characters?
- Syntactic Structures: Do the sentence structures differ from typical human writing patterns?
- Stylistic Nuances: Is there a noticeable difference in writing style, such as formality or personal tone?
When human writing diverges significantly from machine-generated text along these metrics, it signals a potential blend of synthetic authorship.
The Role of Data Science in AI Detection
If you’re feeling your pulse race at terms like “mathematical model” and “data collection,” you’re not alone. Data scientists like Yong Keong Yap are at the forefront of creating and refining AI content detectors. At Ahrefs, where the team is actively building their own AI content detector, the emphasis is on continually gathering qualitative data that reflects varied writing styles. This means their tools grow smarter with each update.
Addressing Future Implications
While current models show promising results, the conversation about AI-generated content detection is not likely to dwindle. As AI implementations become ever more sophisticated, so too must our critical approaches to discern these subtle differences. There are future implications for marketers, SEO professionals, and businesses at large. As AI content becomes increasingly commonplace, the demand for effective detection tools will soar. Understanding these dynamics will enable marketers to navigate the murky waters of content authenticity and integrity.
Common Misconceptions Surrounding AI Content Detectors
There’s a pervasive belief that AI content detection is infallible or that a reliable detection method exists as a one-size-fits-all tool. Unfortunately, this is far from the truth. The reality is that as AI technology evolves, those same models tasked with detecting AI-generated content go through their own evolutionary process. The potential for false positives, or incorrectly identifying human content as AI-generated, remains a pressing concern.
Prospective Trends in AI Content Creation
From a marketing perspective, propelling forward into an AI-rich future generates heated dialogues about regulation, responsibility, and content creation. Ultimately, the key takeaway for marketers is to stay ahead of emerging trends—perhaps developing a unique style that resonates more genuinely with audiences, a strategy that machine-generated content may find challenging to replicate.
As AI technology continues its exponential growth, so must our understanding of it, allowing marketers to capitalize on new tools while maintaining the essence of authentic human communication. Stay curious, stay engaged, and always be prepared for what comes next in the world of AI!
Write A Comment